标注数据是一个费时费力的任务,本文介绍了如何使用 MMDetection 中的 RTMDet 算法联合 Label-Studio 软件进行半自动化标注。具体来说,使用 RTMDet 预测图片生成标注,然后使用 Label-Studio 进行微调标注,可以参考此流程和方法,将其应用到其他领域。
- RTMDet:RTMDet 是 OpenMMLab 自研的高精度单阶段的目标检测算法,开源于 MMDetection 目标检测工具箱中,其开源协议为 Apache 2.0,工业界的用户可以不受限的免费使用。
- Label Studio 是一款优秀的标注软件,覆盖图像分类、目标检测、分割等领域数据集标注的功能。
本文将使用猫猫数据集的图片,进行半自动化标注。
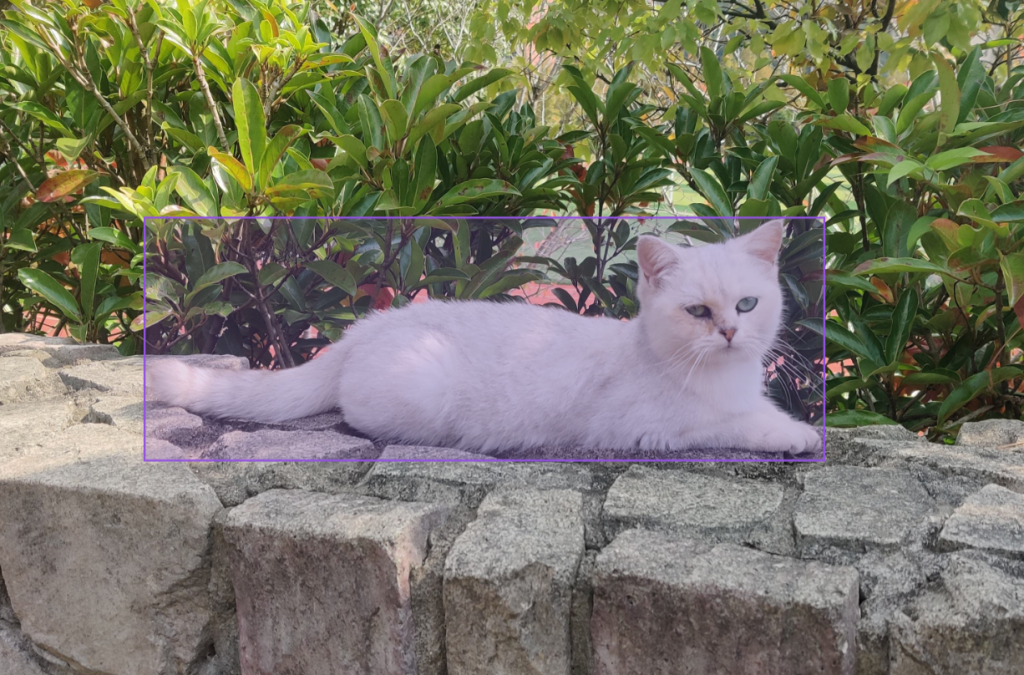
本地环境配置教程:
服务器环境配置教程:
Docker 快速安装 Label-Studio:
环境配置
首先需要创建一个虚拟环境,然后安装 PyTorch 和 MMCV。在本文中,我们将指定 PyTorch 和 MMCV 的版本。接下来安装 MMDetection、Label-Studio 和 label-studio-ml-backend,具体步骤如下:
创建虚拟环境:
conda create -n rtmdet python=3.9 -y conda activate rtmdet
安装 PyTorch
# Linux and Windows CPU only pip install torch==1.10.1+cpu torchvision==0.11.2+cpu torchaudio==0.10.1 -f https://download.pytorch.org/whl/cpu/torch_stable.html # Linux and Windows CUDA 11.3 pip install torch==1.10.1+cu113 torchvision==0.11.2+cu113 torchaudio==0.10.1 -f https://download.pytorch.org/whl/cu113/torch_stable.html # OSX pip install torch==1.10.1 torchvision==0.11.2 torchaudio==0.10.1
安装 MMCV
pip install -U openmim mim install "mmcv>=2.0.0" # 安装 mmcv 的过程中会自动安装 mmengine
安装 MMDetection
git clone https://github.com/open-mmlab/mmdetection cd mmdetection pip install -v -e .
安装 Label-Studio (或者使用docker快速搭建 Label-Studio )和 label-studio-ml-backend
# 安装 label-studio 需要一段时间,如果找不到版本请使用官方源 pip install label-studio==1.7.2 pip install label-studio-ml==1.0.9
下载rtmdet权重
cd path/to/mmetection mkdir work_dirs cd work_dirs wget https://download.openmmlab.com/mmdetection/v3.0/rtmdet/rtmdet_m_8xb32-300e_coco/rtmdet_m_8xb32-300e_coco_20220719_112220-229f527c.pth
启动服务
启动 RTMDet 后端推理服务:
cd path/to/mmetection label-studio-ml start projects/LabelStudio/backend_template --with \ config_file=configs/rtmdet/rtmdet_m_8xb32-300e_coco.py \ checkpoint_file=./work_dirs/rtmdet_m_8xb32-300e_coco_20220719_112220-229f527c.pth \ device=cpu \ --port 8003 # device=cpu 为使用 CPU 推理,如果使用 GPU 推理,将 cpu 替换为 cuda:0
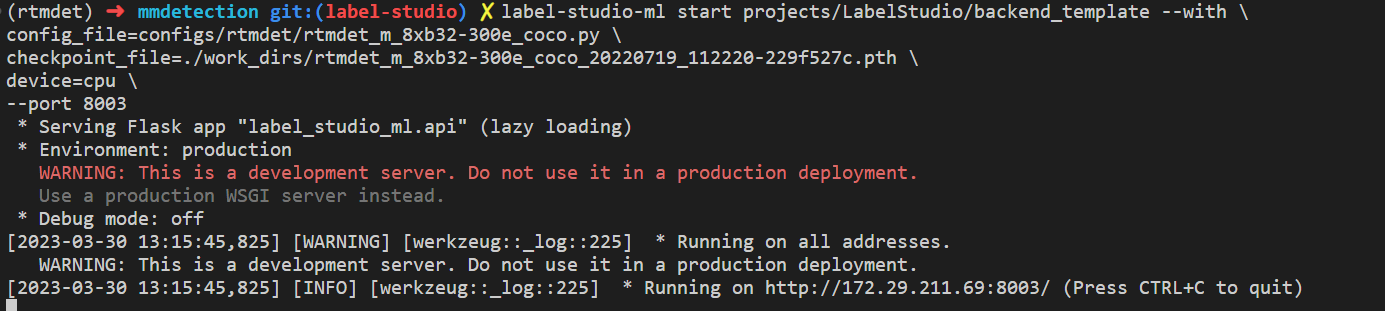
此时,RTMDet 后端推理服务已经启动,后续在 Label-Studio Web 系统中配置 http://localhost:8003 后端推理服务即可。
现在启动 Label-Studio 网页服务:(或者使用docker快速搭建 Label-Studio )
label-studio start
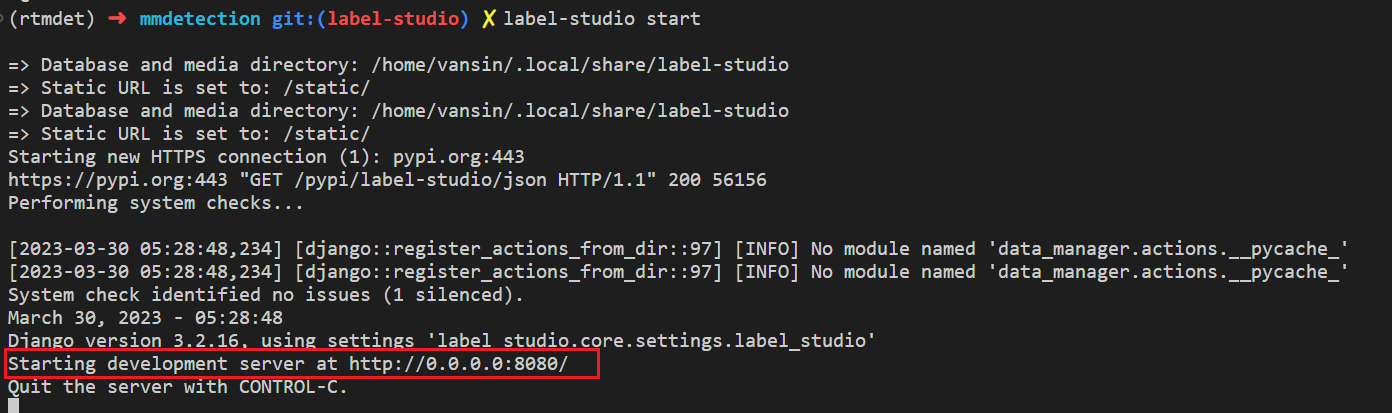
打开浏览器访问 http://localhost:8080/ 即可看到 Label-Studio 的界面。
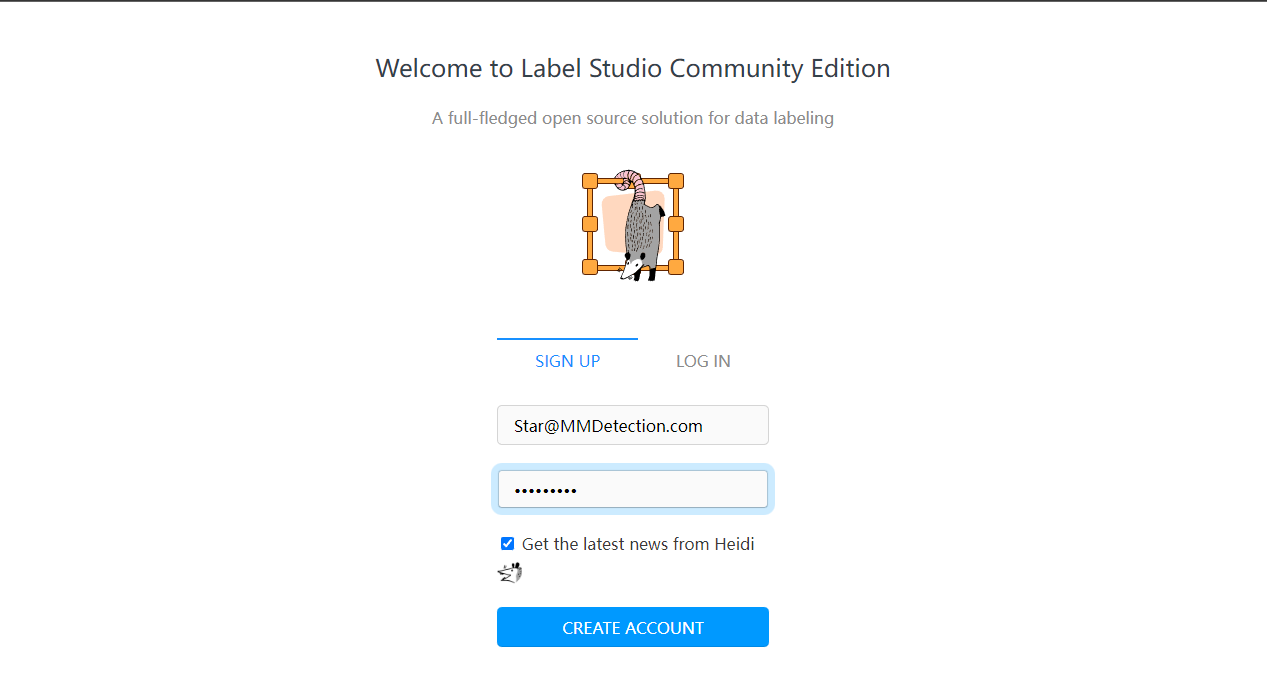
我们注册一个用户,然后创建一个 RTMDet-Semiautomatic-Label 项目。
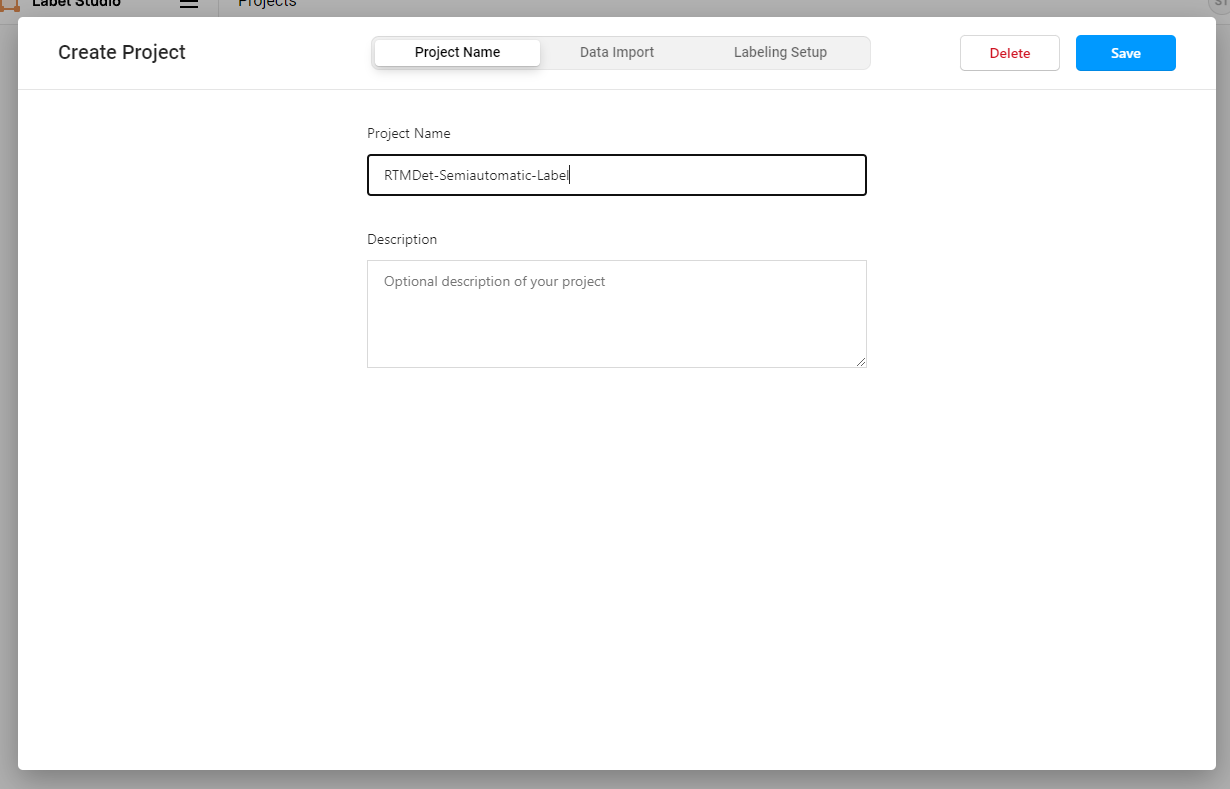
我们通过下面的方式下载好示例的喵喵图片,点击 Data Import 导入需要标注的猫图片。
cd path/to/mmetection mkdir data && cd data wget https://download.openmmlab.com/mmyolo/data/cat_dataset.zip && unzip cat_dataset.zip
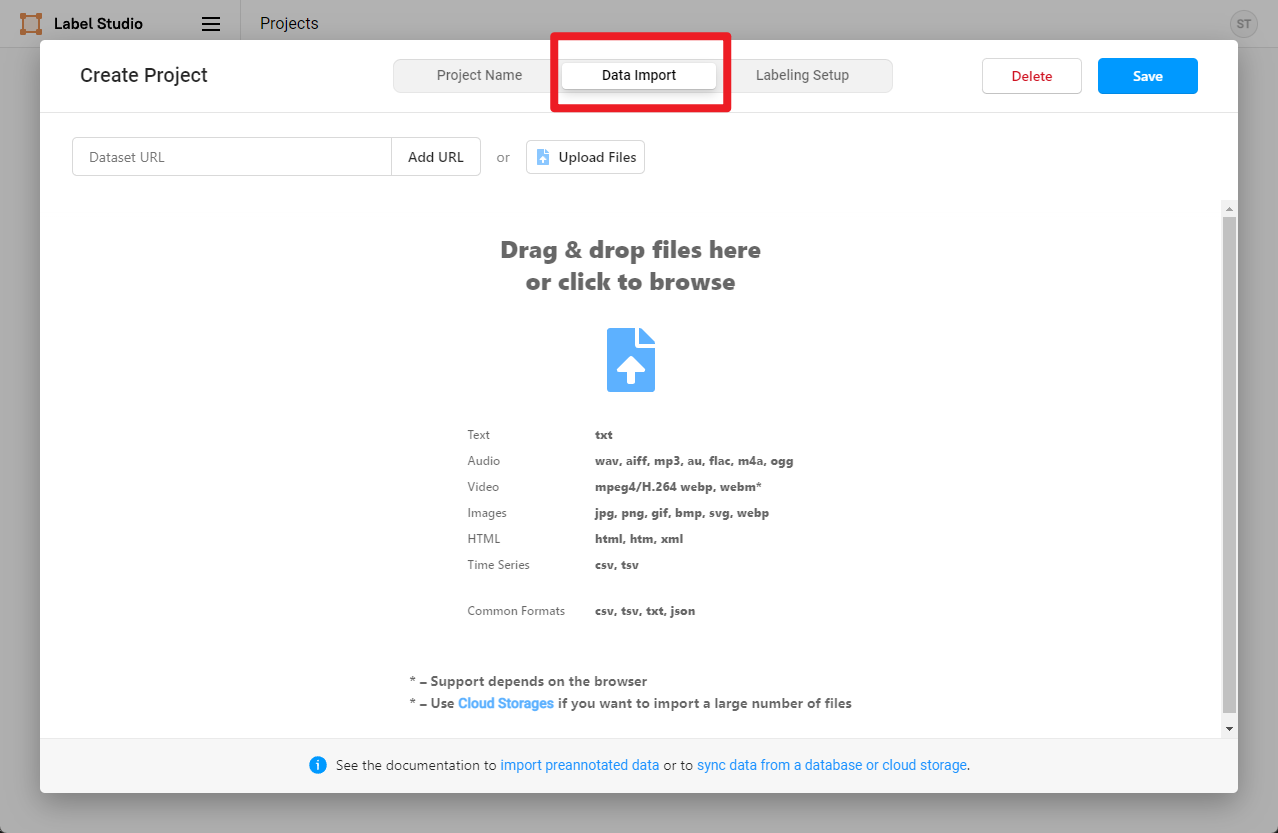
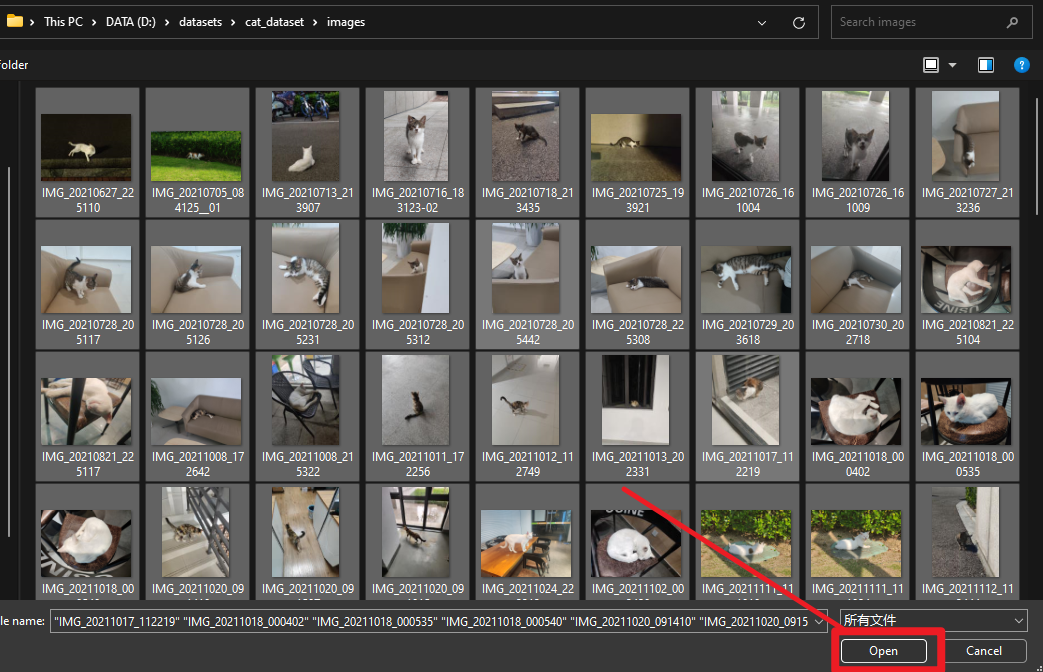
然后选择 Object Detection With Bounding Boxes 模板
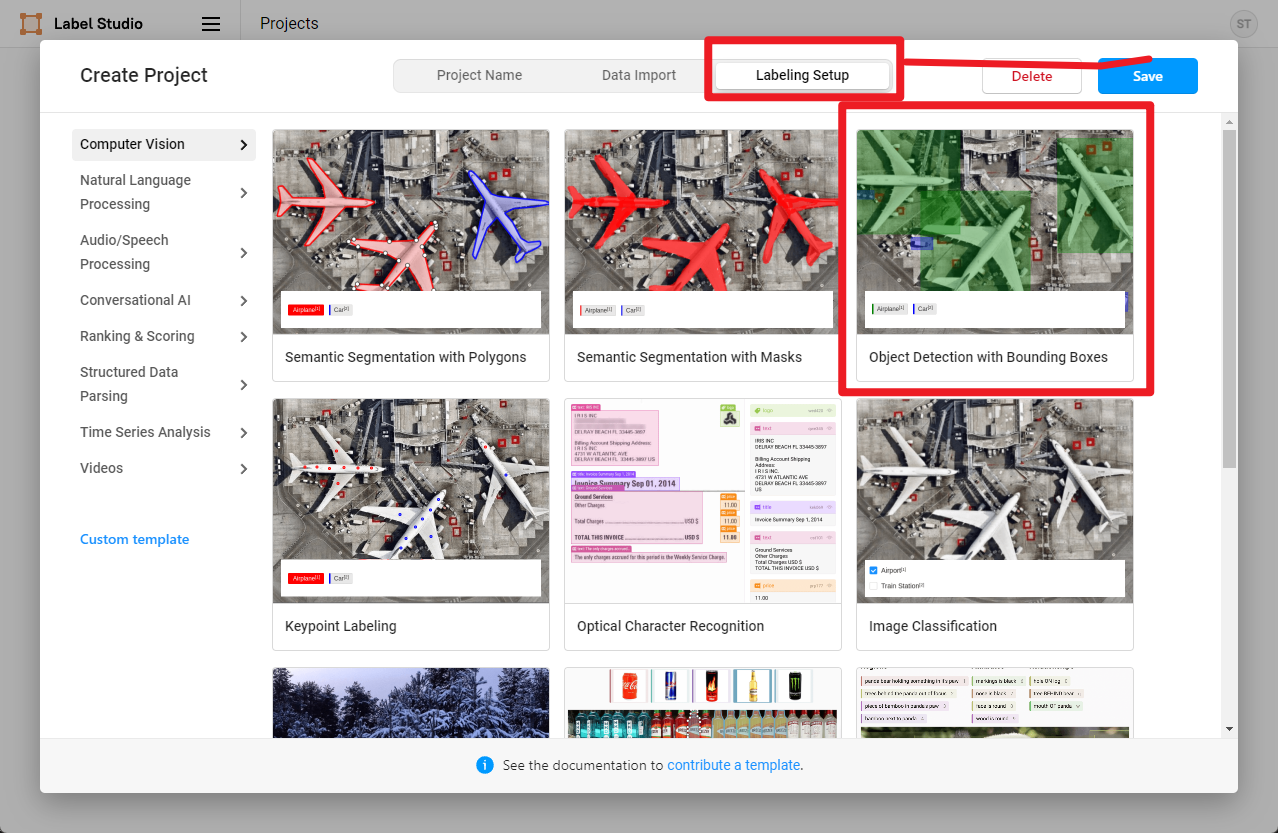
airplane apple backpack banana baseball_bat baseball_glove bear bed bench bicycle bird boat book bottle bowl broccoli bus cake car carrot cat cell_phone chair clock couch cow cup dining_table dog donut elephant fire_hydrant fork frisbee giraffe hair_drier handbag horse hot_dog keyboard kite knife laptop microwave motorcycle mouse orange oven parking_meter person pizza potted_plant refrigerator remote sandwich scissors sheep sink skateboard skis snowboard spoon sports_ball stop_sign suitcase surfboard teddy_bear tennis_racket tie toaster toilet toothbrush traffic_light train truck tv umbrella vase wine_glass zebra
然后将上述类别复制添加到 Label-Studio,然后点击 Save。
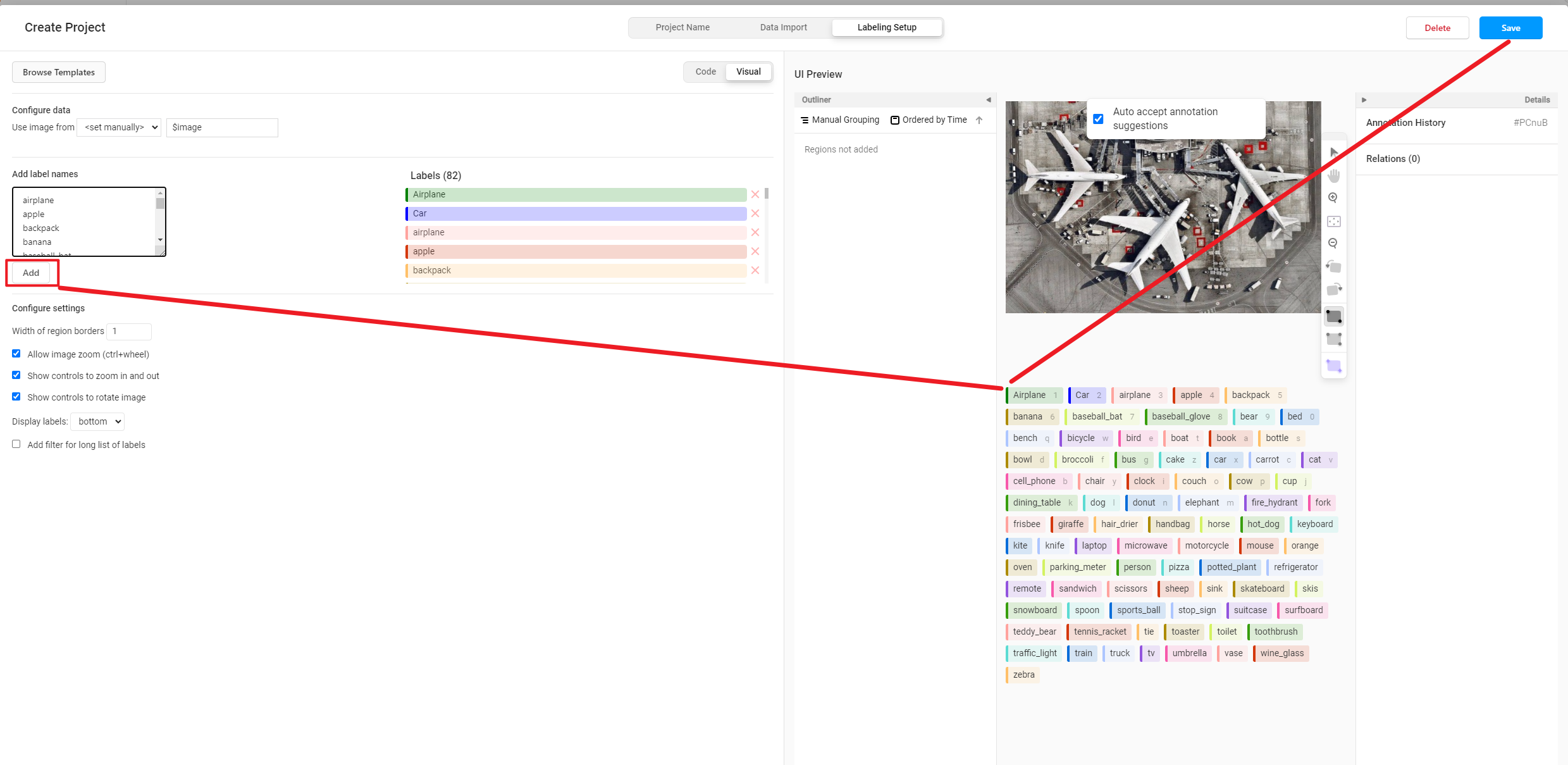
然后在设置中点击 Add Model 添加 RTMDet 后端推理服务。
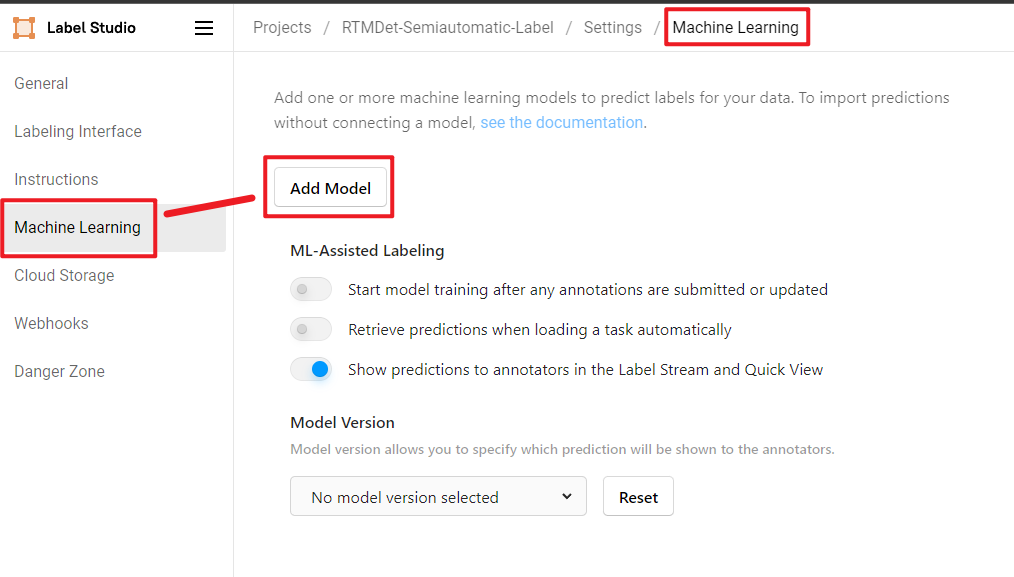
点击 Validate and Save,然后点击 Start Labeling。
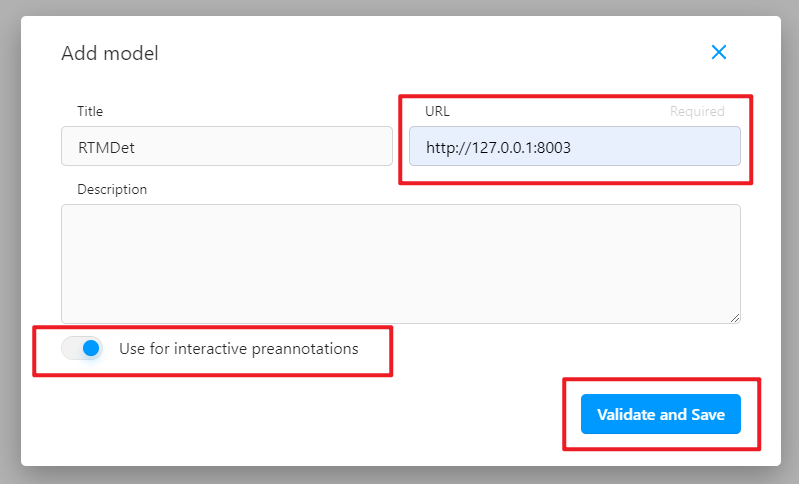
看到如下 Connected 就说明后端推理服务添加成功。
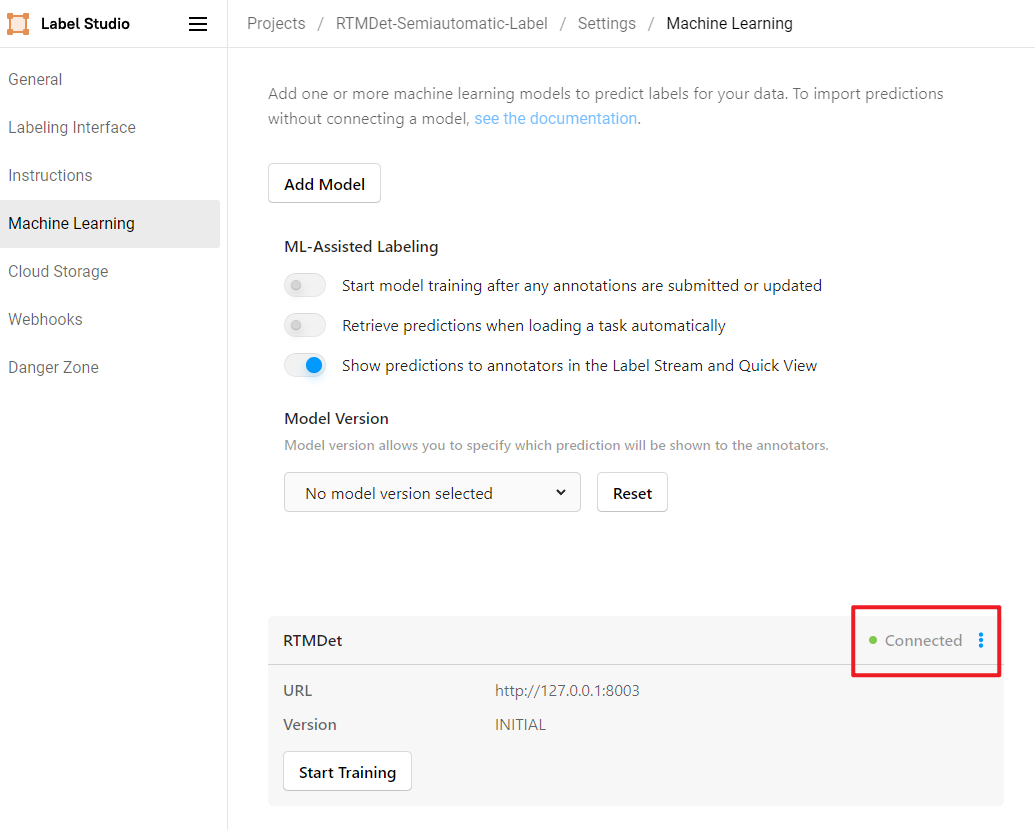
开始半自动化标注
点击 Label 开始标注
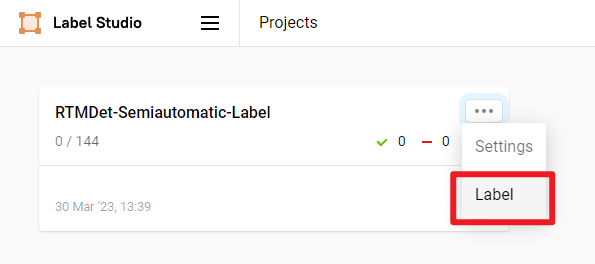
我们可以看到 RTMDet 后端推理服务已经成功返回了预测结果并显示在图片上,我们可以发现这个喵喵预测的框有点大。
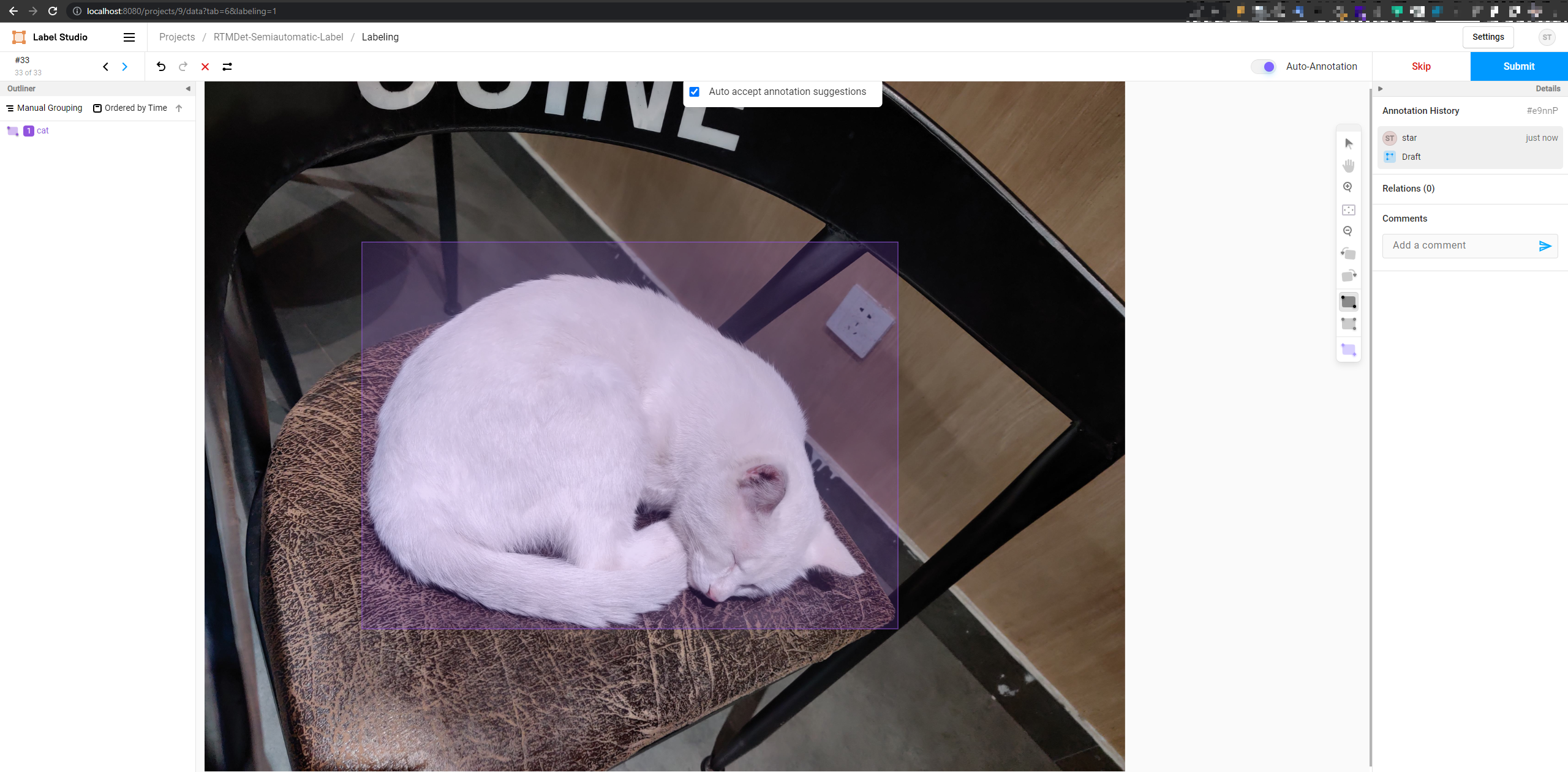
我们手工拖动框,修正一下框的位置,得到以下修正过后的标注,然后点击 Submit,本张图片就标注完毕了。
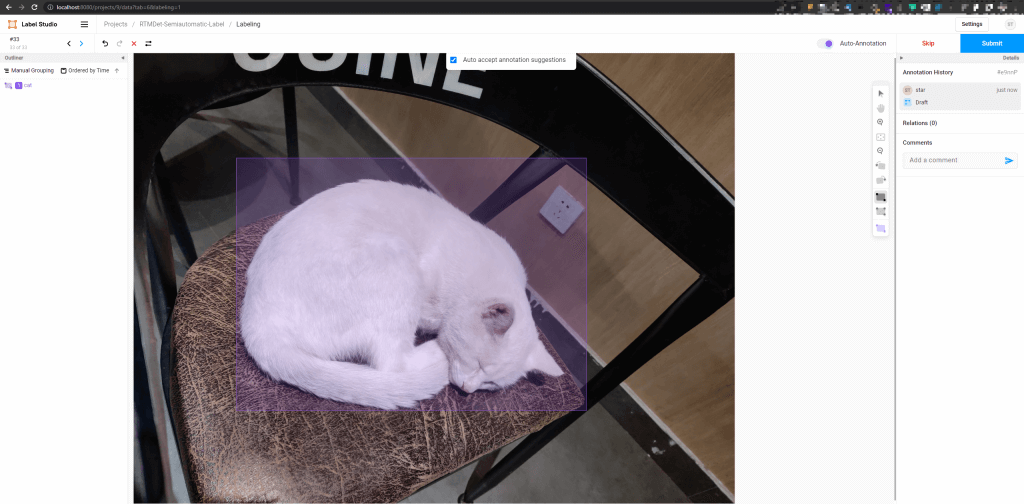
我们 submit 完毕所有图片后,点击 exprot 导出 COCO 格式的数据集,就能把标注好的数据集的压缩包导出来了。
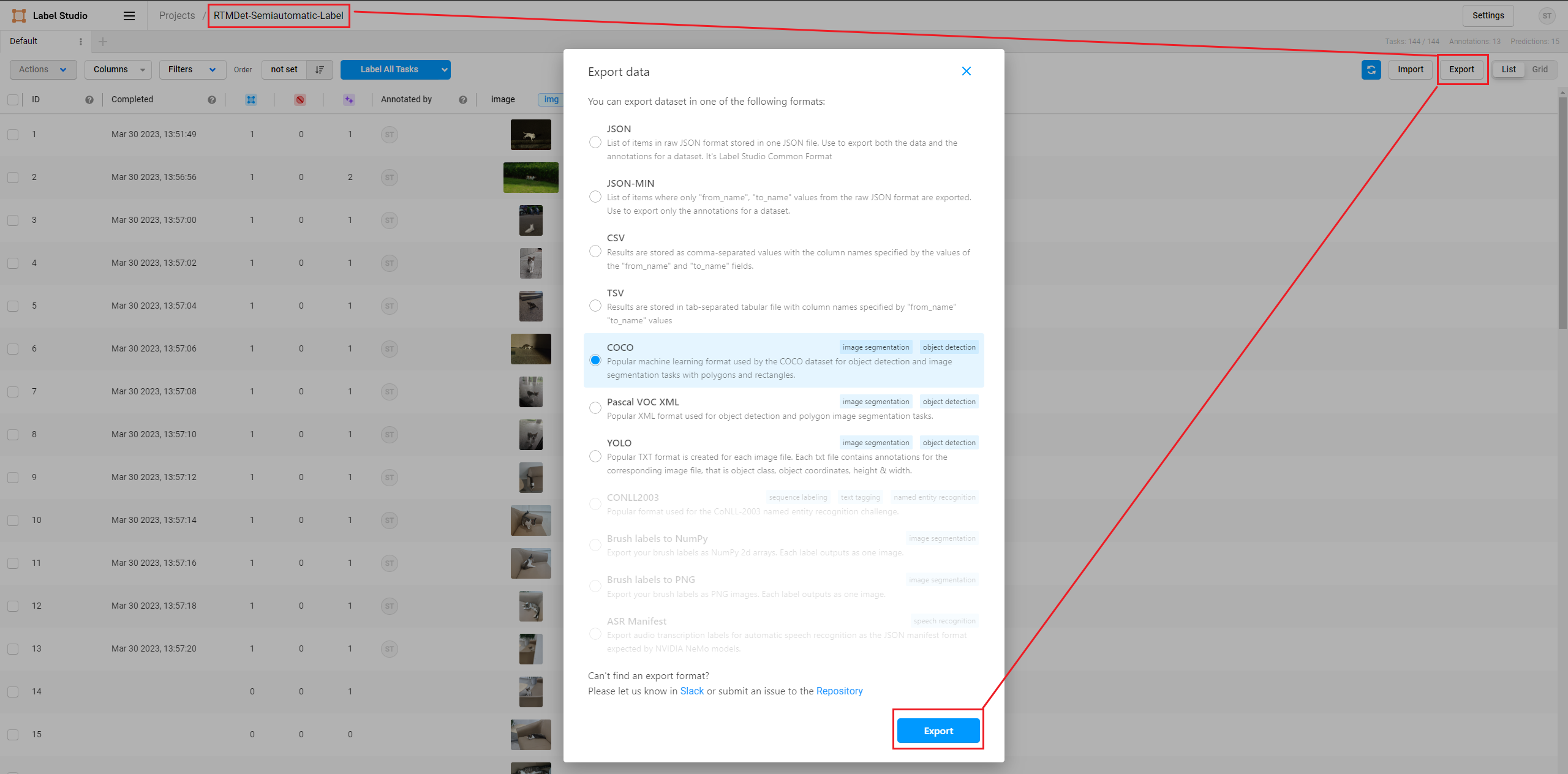
用 vscode 打开解压后的文件夹,可以看到标注好的数据集,包含了图片和 json 格式的标注文件。
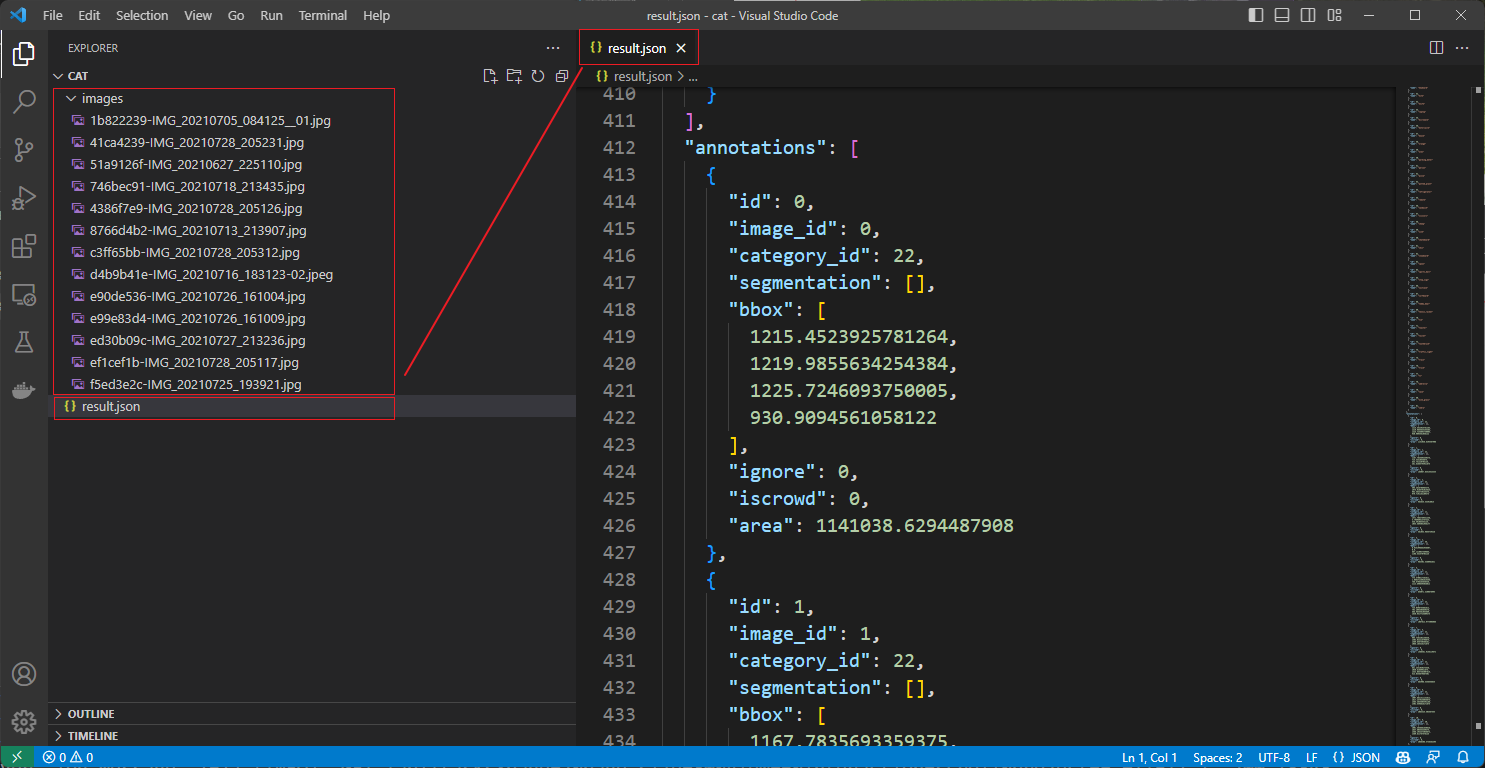
到此半自动化标注就完成了,我们可以用这个数据集在 MMDetection 训练精度更高的模型了,训练出更好的模型,然后再用这个模型继续半自动化标注新采集的图片,这样就可以不断迭代,扩充高质量数据集,提高模型的精度。
使用 MMYOLO 作为后端推理服务
如果想在 MMYOLO 中使用 Label-Studio,可以参考在启动后端推理服务时,将 config_file 和 checkpoint_file 替换为 MMYOLO 的配置文件和权重文件即可。
cd path/to/mmetection label-studio-ml start projects/LabelStudio/backend_template --with \ config_file= path/to/mmyolo_config.py \ checkpoint_file= path/to/mmyolo_weights.pth \ device=cpu \ --port 8003 # device=cpu 为使用 CPU 推理,如果使用 GPU 推理,将 cpu 替换为 cuda:0
踩坑记录:后端推理模型必须和前端Label-Studio搭建在一台服务器上,因为后端某处调用Label-Studio图片数据使用的是localhost,跨服务器会导致http request error,目前没有找到修改这个localhost的方法。
参考:
https://mmdetection.readthedocs.io/zh-cn/latest/user_guides/label_studio.html